AI & Customer listening: is NPS outdated?
AI & Social listening: continuous analysis of online customer feedback: the key to a secure experience, closest as possible to customer's needs.
As many businesses and agencies are reopening in the context of deconfinement, health concerns, and the issue of customer satisfaction are more than ever at the heart of business concerns. Indeed, it is not only a question of successfully passing the test of reopening by meeting customer expectations in terms of hygiene, barrier gestures, and the safe availability of products and services; but also of making up for part of the loss of earnings related to the period of confinement.
Rapidly detecting health hazards, continuously monitoring customer satisfaction and dissatisfaction drivers, analyzing the impact of measures taken; these are all actions for which datascience makes it possible to speed up processing and respond in almost real-time, where conventional barometers find their limits.
Our Datascience Sia Partners team has developed an Artificial Intelligence (AI) solution to collect, analyze and monitor the level and causes of customer satisfaction/dissatisfaction, to compare them within the same brand between several outlets, or with the competition, and to build new indicators of the company's performance in terms of customer experience. A way to develop customer listening and its impact on the company's offers and processes without over-solicitation of the customer with multiple surveys. This solution also makes it possible to detect weak signals (e.g. health risks in food retailing and new customer expectations in terms of prevention).
The contribution of AI as a source of customer feedback
Before any customer experience transformation program, it is crucial for companies to offer at least quality service. The first level of maturity in customer experience management, according to Forrester, is to "fix the experience”. This means adopting "best practices that lead to identifying, fixing the experience, and measuring results”. This was traditionally done in companies through hot or cold surveys, which made it possible to calculate an NPS (Net Promoter Score).
The NPS is an indicator that reflects a customer's propensity to promote the brand or service, based on their response to the following question: "On a scale of 0 to 10, how likely is it that you would recommend this product or service to a friend"? Customers who gave a score of 0 to 6 are detractors, 7 to 8 are neutral, 9 to 10 are promoters. The NPS is calculated by subtracting the percentage of detractors from the percentage of promoters.
In fact, these questionnaires are an effective way to obtain indicators on the quality of the customer experience and to collect verbatim. In addition to satisfaction barometers, analysis of the reasons for complaints, internal feedback collected by the company, etc., these questionnaires are an effective way of obtaining indicators on the quality of the customer experience and collecting verbatim feedback.
We are convinced that open data could bring significant added value to customer care. This is why our Datascience team has developed a solution that capitalizes on online customer reviews, offering the possibility of collecting spontaneous customer reviews on a large scale.
Indeed, as the number of comments increases exponentially for all distribution networks, this tool allows a direct evaluation of the brands, services, and products offered at different levels: general, product, service, personnel, organization, operations, etc.; as well as a comparison of their performance (over a given geographic area, at the network level, with the competition, etc.). These customer opinions cross-referenced with our AI and the tool's various functionalities can thus be used as a driver for continuous improvement of the customer experience and innovation, at several levels.
The different levels of analysis allow us to address the pain points of the customer experience at the point of sales/branch level.
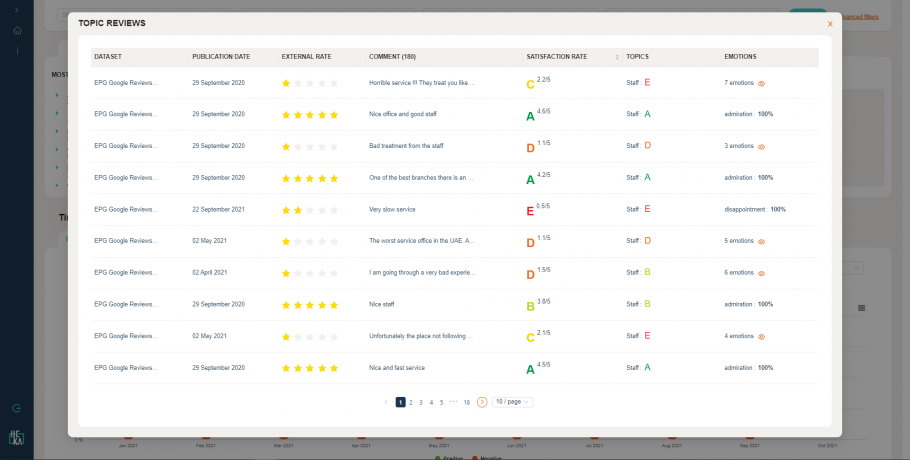
This tool allows not only a precise and real-time measurement of customer satisfaction on the themes followed by the company but also to highlight weak signals on themes not followed by the company. This functionality is part of our "Consulting for Good" project for an AI to detect ethical risks and inappropriate attitudes of employees and companies. For example, the analysis of verbatims can reveal problems of accessibility, or discrimination in agencies for example.
This analysis also makes it possible to highlight areas for improvement that must be taken into account as a matter of urgency: this may be the case for opinions concerning the behavior of an employee or the cleanliness of the agency. By detecting them quickly and easily, it is possible to correct them immediately and have a significant impact on customer satisfaction.
Artificial Intelligence then contributes to several levels of customer listening:
- Upstream on the obtaining of information, the finesse of analysis and interpretation.
- On the preparation of remedial actions to improve the customer experience. Machine Learning enables the correlation between the pain point and the corrective action to predict the impact of the measure taken on the NPS. Through testing, the AI must enable the definition of the right combination of corrective actions to be implemented depending on the occurrence of pain points. This functionality proposed by the Sia Partners solution and to be co-constructed with our customer's data enables us to define more relevant and precise customer experience improvement projects.
- Downstream on the measurement of corrective actions on customer satisfaction
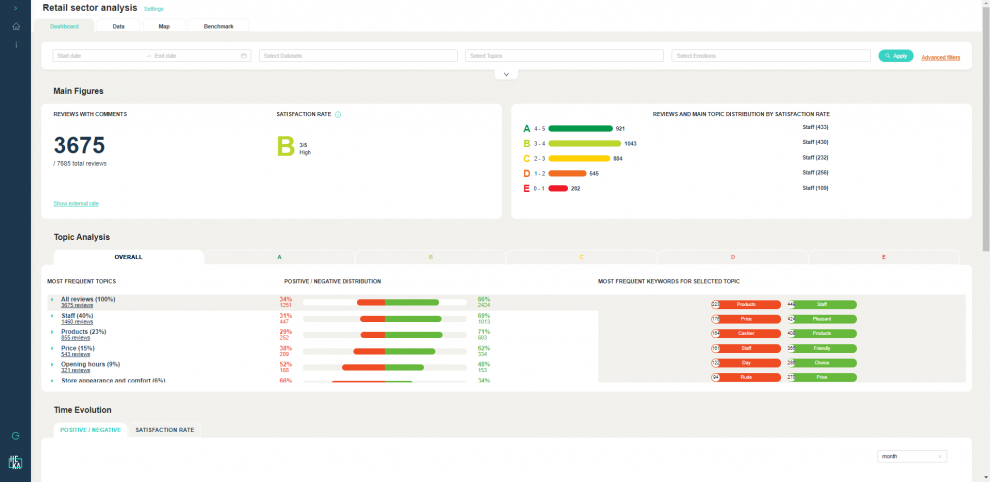
Better understand pain points, their roots, and their cost in terms of customer satisfaction
The customer experience will remain the main differentiator for brands, ahead of price, or product. The studies to support this thesis have been going on for several years now. A study conducted by American Express, for example, tells us that the average consumer is willing to pay up to 14% more for better service in stores. This highlights the real business interest in putting the experience at the heart of the concerns.
In addition to the security aspects related to the deconfinement period, Sia Partners and Potloc conducted a study in April 2020 with 100,000 French consumers to better understand the impact of the Covid-19 crisis on their expectations of brands:
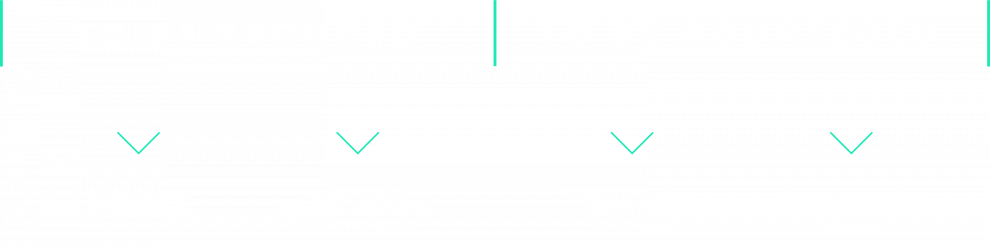
If digital and phygital have been the stars of the last few years for the original solutions they bring to these "must-have '' experiences, and while we were predicting them a slow and painful death, physical stores are still as attractive as ever, especially as they reinvent themselves. By relying on innovations based on experience and using emotion as a lever of attraction, they are regaining their credibility with consumers, who are sometimes disillusioned by self-care tools.
However, if this is a relevant trend when we talk about FMCGs, ready-to-wear stores, luxury boutiques, etc., it is probably a little less relevant if we look at bank branches, post offices, car rental agencies, etc.
Most customer satisfaction analyses simply track the evolution of the NPS score along simple axes: over time, by region. We have chosen to go further by crossing social, demographic, geographical, cadastral, etc. variables to identify the factors influencing the score and customer comments.
To do this, our tool is based on Natural Language Processing (NLP), which enables the analysis and automatic processing of textual content and to extract the necessary information from it.
The NLP thus allows us to :
- Group comments by theme
- Isolate the points of pain, and to qualify the extent of the problem thanks to their frequency in the comments.
- Highlight the subjective (customer experience) and objective factors influencing overall satisfaction.
- Make the data speak by correlating them with socio-economic, geographical, weather and company diary data in order to go back to the root causes of each pain point.
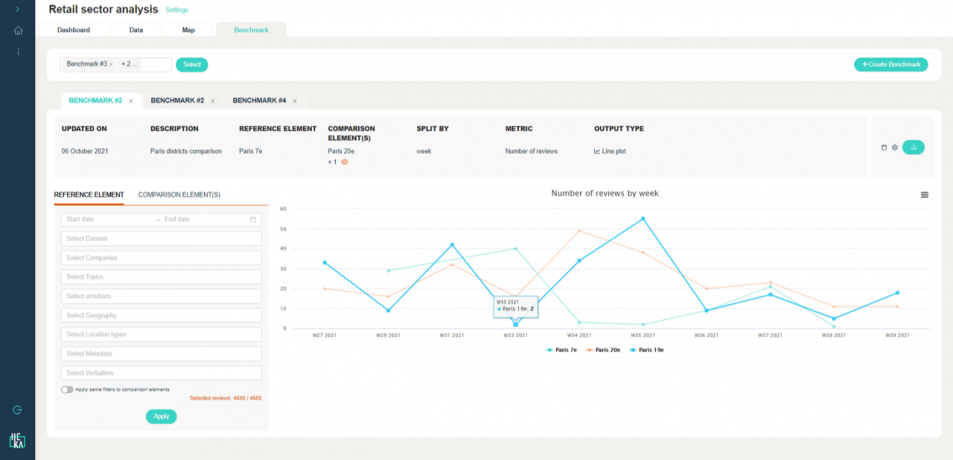
Our data scientists have developed algorithms for modeling customer feedback scores to accurately quantify the impact of each variable on customer satisfaction. As an output, it is, therefore, possible to consult the different reasons and factors of satisfaction/dissatisfaction.
This type of approach allows us to determine the "cost on satisfaction" of each pain point but also the potential gains obtained in case of resolution. It is thus possible to prioritize the actions to be undertaken in order to resolve the most problematic points first. Taking all the work together, the elaboration of a prioritized action plan must consider the following aspects:
- The most problematic dysfunctions (thanks to our model)
- The costs and means to be implemented for their resolution (via root cause analysis)
Acting on satisfaction and operational efficiency
By correlating these data with those of traditional repositories (CRM, CEM...), data science highlights objective and quantified trends that guide the company's strategic choices.
Ai allows to:
- Measure changes according to the actions taken by the company.
- Compare the results of the sales forces according to customer feedback on their portfolio of agencies/points of sale.
- Encourage the sharing of best practices within the distribution network.
AI accelerates the analysis of customer behaviors and needs, a boon for brands that can arm themselves with this near real-time information to create a stronger bond with the consumer and optimize the performance of their physical network.
For example, in-depth knowledge of the customer is what enables UMPQA Bank to offer a different branch experience that is valued by the customer: a project that results in a redefinition of the job of customer advisers and a radical overhaul of the function given to the bank branch. And it's working, as brand affinity, customer satisfaction and turnover have increased significantly.
Once the weak points in the customer experience have been identified (these pain points add to a library of attrition causes maintained by our Customer Experience practice).
Sia Partners assists its clients in solving key challenges to deliver a superior and differentiating customer experience and thus limit costly churn at all levels of business.
Our AI solution enables us to define the actions to be taken to remedy the pain points identified from a library of corrective actions established by our Customer Experience practice (CSR training, soft skills, agency redesign, adjusted schedules, installer behavior, returns processing, etc.). Indeed, good management of customer dissatisfaction nowadays enables up to 70% of dissatisfied customers to become loyal again, and therefore significantly improves your customer relationship (Source: LeeRessources).
What about tomorrow? Towards a predictive NPS
Artificial intelligence allows the development of analytical capabilities to build "predictive NPS", which offers several use cases:
- First of all, AI makes it possible to analyze, understand, and predict the characteristics of customer profiles. Without sending a survey to a new customer, it will thus be possible to predict the most important drivers of his satisfaction and to predict what his status would have been (Promoter, Neutral or Detractor), based on the responses of other customers with similar profiles or "lookalikes". This knowledge of the critical drivers of satisfaction should guide the prioritization of action plans to correct irritants. Furthermore, it helps to avoid over-solicitation of customers with surveys and to better manage marketing pressure.
- Once the AI has detected what a customer's profile looks like, it becomes possible to predict his behavior. For example, if a customer has responded to an NPS survey as a Detractor, then the AI can alert the company that lookalikes customers are at risk of becoming Detractors even before they have responded to a survey. In addition to proactive risk detection, predictive NPS can also detect passive Detractors, who do not express dissatisfaction but still present a high risk of churn.
- Finally, when a customer expresses dissatisfaction or when irritants are detected, the AI can predict the best action plan that optimizes the chances of correcting the irritant and re-satisfying the customer.
Some companies have already started to use predictive analytics: this is the case of Uber's Chinese competitor - Didi. Very advanced in the analysis of the context, the company no longer questions its users to find out their satisfaction rating. This rating is automatically calculated according to travel time, delays, and Didi's knowledge of the customer. Predictive analysis is used here to determine satisfaction on 100% of the trips.
To successfully implement these use cases, companies need to develop strong analytical capabilities:
- First of all, companies need to develop operational efficiency quick-wins per channel: simplify and automate processes, optimize customer orientation according to their profile and contact pattern towards the optimal channel, including self-care.
- Then, companies must develop longer-term capabilities to build a cross-channel vision and anticipate changes: collect and consolidate customer feedback on the different channels, analyze critical episodes during customer journeys with the irritants and their underlying causes, integrate customer knowledge from surveys into CRM tools and processes, implement an agile operating model to drive customer satisfaction.
Our Marketing & Customer Experience and Datascience teams support many key accounts in the implementation of satisfaction industrialization programs and will be able to assist you in the implementation of innovative solutions generating measurable results.