Our SmartDataQuality.ai solution to quantify data quality issues
Data is the keystone of performance and the key factor of our activities on a global scale; it is constantly covering new domains, extending to new applications and representing an ever increasing weight.
In this context, it is crucial to understand the stakes involved and to have the right tools.
Data is ubiquitous in today's world and its global volume continues to grow every year and has reached 50 zettabytes in 2020, which is equivalent to 6 million 2-hour high definition movies. This impressive figure shows the importance of data today, especially for companies that want to become data-driven and incorporate data into their decision making. To keep up with this increase in volume, data quality is an essential prerequisite for the efficient use of company data, and more broadly for any data science project.
At a time when companies are increasingly turning to a data-driven strategy, Heka.ai proposes a series of two articles: the first article will seek to quantify the data quality problem in a pragmatic way on the basis of our partners' data studied with SmartDataQuality.ai. The second article will advocate an approach to address the challenge of data quality faced by companies.
Quantifying the issue of data quality with our SmartDataQuality.ai solution
The projects carried out with our clients on their data quality have led us to imagine a generic solution to address this challenge common to all sectors. This is the genesis of our solution: SmartDataQuality.ai. Our tool allows us to :
- Detect duplicates within your databases, mainly false duplicates. As an illustration: customer databases are made up of a number of duplicates that are difficult to spot, such as "Mathieu Martin" and "Martin Mathieu". By leveraging artificial intelligence methods, our solution is able to identify these duplicates with an associated confidence index.
- Enrich databases with external information by intelligently reconciling different repositories. Smart Data Quality is able to enrich customer databases with socio-demographic information (such as median income, age distribution, etc.), information on the address' cadastral parcel (such as the surface area of the land, the living area, etc.). By cross-referencing several sources of information, inconsistencies become more apparent. More generally, database enrichment is a real lever to enable companies to optimize their processes: communications with customers can be more relevant, for example.
- Carry out diagnostics on data quality in a few clicks. For example, SmartDataQuality.ai allows to diagnose the quality of addresses present in a database or the veracity of numerical values.
- Carry out data normalization processes: SmartDataQuality.ai identifies similarities and proposes column normalizations based solely on the analysis of the data file.
Moreover, all these algorithms can be saved in order to analyze their performance, but also to apply them later on to other databases.
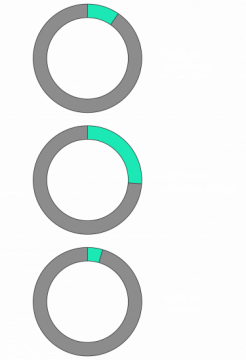
Some key figures regarding data quality:
These indicators may seem abstract, but have a strong operational reality:
- Nearly 10% of outbound communication costs can be reduced by dealing with duplicates in customer databases,
- Nearly a quarter of operational activities, such as a telephone conversation with a customer or a maintenance intervention, can be improved by filling in missing data,
- Good data quality can prevent up to 5% of customer invoices being sent to the wrong address or even containing incorrect information.
In general, this poor data quality is due to human errors: typographical errors, omissions in data entry. It can also be due to poor communication between the different entities of the company, or even to perfectible business processes. These errors lead to significant costs for companies. Studies have shown that 1 to 5% of incorrect data leads to an increase in operational costs up to 8 to 12%. Beyond the costs, poor data quality hinders the company's desire to become data-driven, because it leads to
- Risky or even inappropriate decisions
- Inefficiency of data-driven activities and processes
- Very little confidence in the results obtained
- Missed opportunities
- Lost revenue
In a forthcoming publication, we will discuss how to address this issue in a comprehensive way within the company. If you are also interested in a free diagnosis of one of your databases, please contact us at smartdataquality@sia-partners.com.